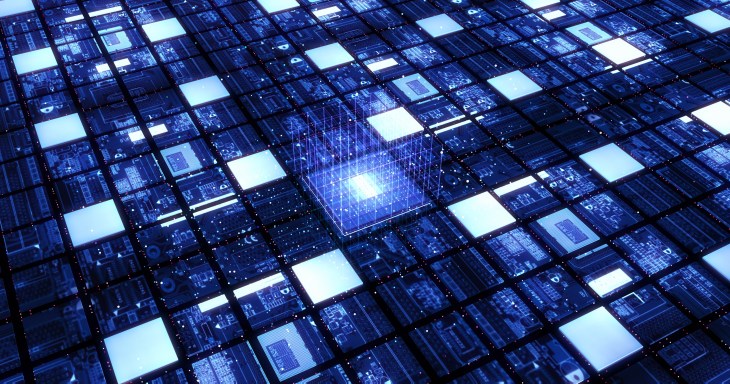
ChatGPT’s launch ushered within the age of huge language fashions. Along with OpenAI’s choices, different LLMs embody Google’s LaMDA household of LLMs (together with Bard), the BLOOM venture (a collaboration between teams at Microsoft, Nvidia, and different organizations), Meta’s LLaMA, and Anthropic’s Claude.
Extra will little doubt be created. In actual fact, an April 2023 Arize survey discovered that 53% of respondents deliberate to deploy LLMs inside the subsequent 12 months or sooner. One strategy to doing that is to create a “vertical” LLM that begins with an current LLM and thoroughly retrains it on information particular to a selected area. This tactic can work for all times sciences, prescription drugs, insurance coverage, finance, and different enterprise sectors.
Deploying an LLM can present a strong aggressive benefit — however provided that it’s completed properly.
LLMs have already led to newsworthy points, resembling their tendency to “hallucinate” incorrect data. That’s a extreme downside, and it could possibly distract management from important issues with the processes that generate these outputs, which will be equally problematic.
The challenges of coaching and deploying an LLM
One difficulty with utilizing LLMs is their large working expense as a result of the computational demand to coach and run them is so intense (they’re not known as giant language fashions for nothing).
LLMs are thrilling, however growing and adopting them requires overcoming a number of feasibility hurdles.
First, the {hardware} to run the fashions on is expensive. The H100 GPU from Nvidia, a preferred alternative for LLMs, has been promoting on the secondary marketplace for about $40,000 per chip. One supply estimated it could take roughly 6,000 chips to coach an LLM corresponding to ChatGPT-3.5. That’s roughly $240 million on GPUs alone.
One other important expense is powering these chips. Merely coaching a mannequin is estimated to require about 10 gigawatt-hours (GWh) of energy, equal to 1,000 U.S. properties’ yearly electrical use. As soon as the mannequin is educated, its electrical energy price will range however can get exorbitant. That supply estimated that the facility consumption to run ChatGPT-3.5 is about 1 GWh a day, or the mixed every day power utilization of 33,000 households.
Energy consumption can be a possible pitfall for person expertise when working LLMs on transportable gadgets. That’s as a result of heavy use on a tool may drain its battery in a short time, which might be a big barrier to shopper adoption.