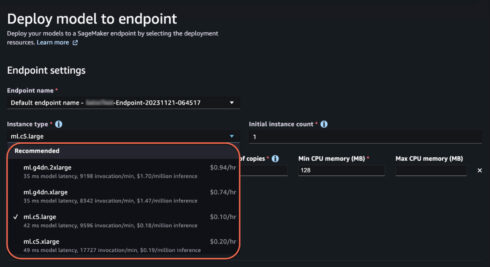
Throughout its AWS re:Invent occasion immediately, AWS introduced a number of updates to Amazon SageMaker, which is a platform for constructing, coaching, and deploying machine studying fashions.
It launched new options which are designed to enhance the mannequin deployment expertise, together with the introduction of latest lessons within the SageMaker Python SDK: ModelBuilder and SchemaBuilder.
ModelBuilder, selects a appropriate SageMaker container to deploy to and captures the wanted dependencies. SchemaBuilder manages the serialization and deserialization duties of inputs and outputs from the fashions.
RELATED CONTENT:
AWS re:Invent Day 1 information
AWS re:Invent Day 2 information
“You need to use the instruments to deploy the mannequin in your native improvement surroundings to experiment with it, repair any runtime errors, and when prepared, transition from native testing to deploy the mannequin on SageMaker with a single line of code,” Antje Barth, principal developer advocate at AWS, wrote in a weblog put up.
SageMaker Studio was additionally up to date with new workflows for deployment, which give steerage to assist select essentially the most optimum endpoint configuration.
SageMaker was additionally up to date with new inference capabilities, which helps scale back deployment prices and latency. The brand new inference capabilities assist you to deploy a number of basis fashions on a single endpoint and management the reminiscence and variety of accelerators assigned to them.
It additionally screens inference requests and robotically routes them based mostly on which cases can be found. In accordance with Amazon, this new functionality will help scale back deployment prices by as much as 50% and scale back latency by as much as 20%.
There have been additionally a number of updates inside Amazon SageMaker Canvas, which is a no-code interface for constructing machine studying fashions. Pure language prompts can now be used when getting ready information.
Within the chat interface, the applying gives quite a lot of guided prompts associated to the database you might be working with, or you’ll be able to provide you with your individual. For instance, you’ll be able to ask it to organize an information high quality report, take away rows based mostly on sure standards, and extra.
As well as, now you can use basis fashions from Amazon Bedrock and Amazon SageMaker Jumpstart. In accordance with the corporate, this new functionality will allow firms to deploy fashions which are designed for his or her distinctive enterprise.
SageMaker Canvas handles all of the coaching and lets you fine-tune the mannequin as soon as it’s created. It additionally gives evaluation of the created mannequin and shows metrics like perplexity and loss curves, coaching loss, and validation loss.